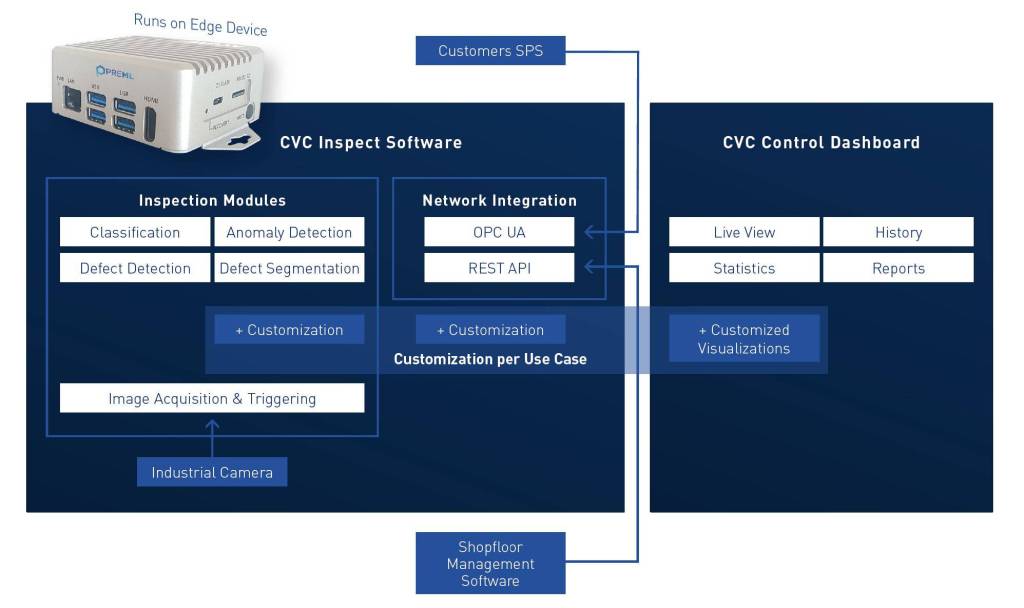
CVC Inspect is a Python-based framework designed to automate visual inspection tasks fast and efficiently. As each visual inspection system comes with its own set of specific requirements, the framework must offer a flexible and modular software architecture for customization. Some modules like image acquisition, different pre-processing operators or communication over specific protocols are the same and can be reused. As a result, each component of the framework can be customized or replaced as needed, while still maintaining an abstract layer for similar functionalities.
At the heart of CVC Inspect are the pipelines, which serve as the abstract main procedures for inspection tasks. Pipelines handle various aspects of the inspection process, from managing different image sources and trigger options to processing the captured images. This abstraction simplifies the implementation of complex inspection workflows, making it easier to configure and manage different inspection tasks. Software hooks in the pipeline are also encluded, which allows the user to add customized modules at various stages of the pipeline, enabling the integration of very specialized algorithms or processing steps. To streamline the setup process, each pipeline is fully configurable through a single file. This allows us to modify inspection setups as requirements evolve by adding or removing different operators to the hooks.
Naturally, the framework contains a lot of different AI based modules as well, such as supervised or unsupervised models, which can easily be exchanged based on a projects requirements. Further, as the framework is python based, we can include the newest state-of-the-art networks effortlessly. One key benefit is, that since the software was fully developed in house and only depends on open source libraries, preML can offer a wide range of licensing options, making sure that machine vision integrators, producing companies and software manufacturers can profit from CVC.
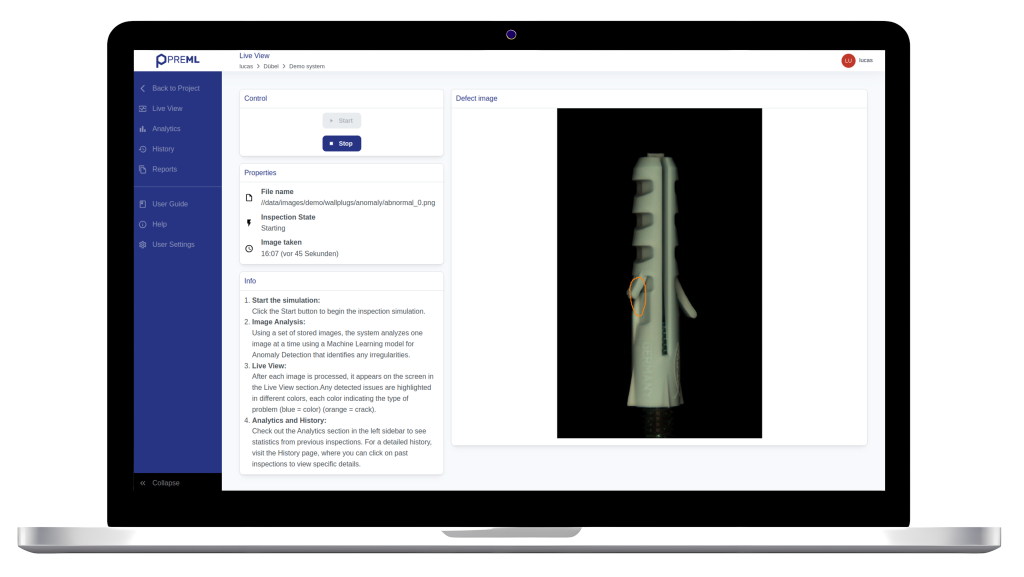
Rapid Real-World Implementation
Additionally, to the CVC Inspect framework, the interactive frontend CVC Control was developed, which seamlessly integrates with CVC Inspect. It is also easy to use for production employees, while maximizing the value of analytics and documentation for quality management. First of all, it is used to visually presents found defects in a modern styled web frontend. Additionally, customers can change specific parameters, collect annotations in real-time from the production site or filter defects with different thresholds.
The design behind CVC Inspect allows to rapidly develop a highly customized inspection system for a wide range of tasks. As it works independently of the product material, size or geometry, the same system can also be used to inspect different types of products with small adjustments. In the real world, this means the same basic software is used for inspecting large concrete parts in difficult environments such as tunnels, as well as in a manual station for inspecting extruded aluminium parts for the automotive industry. However, the user’s hooks might be very different, solving very individual needs of customers. One uses a complex software-based trigger, which is based on an AI tracking algorithm, while another uses a simple TTL signal to trigger the image acquisition. The most unique modules might be an extraction of individual surfaces of an object to project them onto a 3D model for visualization, or training data enrichment with synthetic images. Nevertheless, with each implemented use case, the framework extends, and more modules are available for future use cases.
Location: Lahr/Black Forrest (Germany)
Year founded: 2022
Founder(s): Lucas Steinmann,
David Fehrenbach,
Jonas Fehrenbach
No. of employees: 7
Commercial status: revenue
preML GmbH
Location : Lahr/Black Forrest (Germany)
Year founded: 2020
Founders: Lucas Steinmann, David Fehrenbach, Jonas Fehrenbach
No. of employees: 7
Commercial status: revenue