Hall: It really does depend on the scale of the solution. From a high level perspective, for many years large companies have developed their own clusters for in-house computing. But regarding smaller companies, they are looking at cloud computing solutions because of cost. In that context, where cloud solutions fit they are finding homes. I don’t think that that has to be limited to training. In some cases inference or statistical reporting, quality metrics, production metrics, reporting of all of that data makes a lot of sense in in the cloud. So it can go both ways, but it really depends on the specific use case.
Diani: We are speaking about AI application for machine vision but in my experience AI always has to be combined with the standard tools for machine vision. So I believe that mix of cloud computing and localized computing can solve most of the applications. The learning for sure is a phase in which you need a lot of power but there are small CPUs with a lot of power already available. But I still believe that has to be mixed together with the standard tools of machine vision. So combined tools, local and cloud could be the best solution in the near future.
Where do you see the main benefits of cloud based solutions for automation related vision tasks? What barriers are there to overcome to get customer acceptance for those solutions?
Myakov: Privacy laws and concerns are a big problem. When you transfer data from the edge to the cloud, it actually opens up your data for other risks as well, like theft or copying. But not all data is privacy tricky and yet we have to take the regulatories into account when designing our solutions.
Hall: There is a tendency within the industry to believe that edge computing or on premises computing is is more secure. Maybe because it doesn’t add the element of the transfer to the cloud. However, there is a bit of a fallacy here. In the context of devices which developers believe are to be used in a closed network, they decide not to take advantage of many of the security features that are available in modern devices. So I think the problem with security is not one of edge versus cloud as it is the overall thinking of the developer when they consider what it is exactly that they’re going to implement, whether it’s an edge device or a cloud connection.
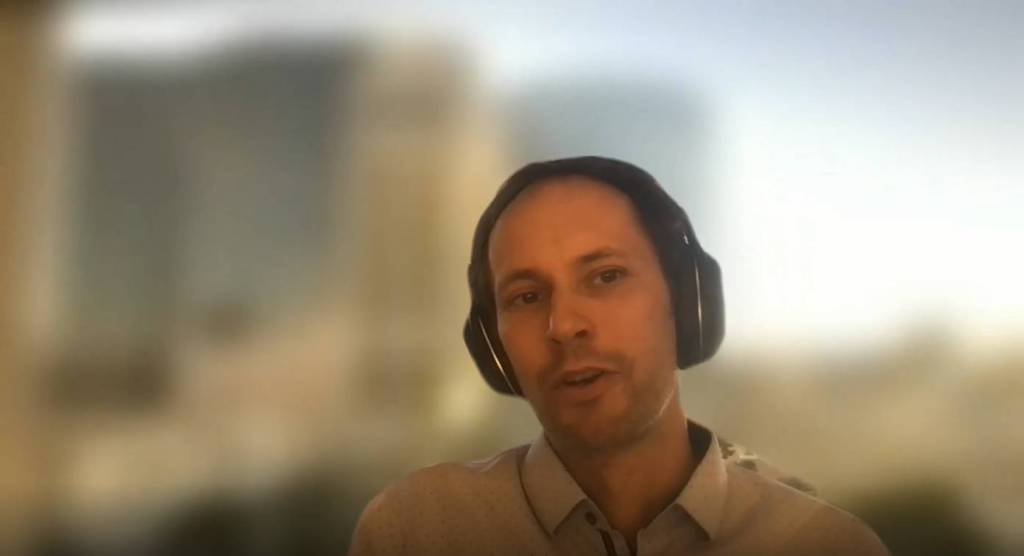
Will Software-as-a-Service be sold more than vision systems and components in the future? And who will offer the new vision systems?
Metzner: The question in the end is who will build the solution and who is offering and who is integrating the solution? A solution consists of many different components, so it’s a broad ecosystem in which we all need to work together.
Myakov: Different companies have different specialties and I don’t think that we are gonna see one to rule them all in the near future. There are some niche examples when such power is concentrated in one hand and but there are very few of those. But typically ecosystems are multilayered: you evolve and you focus on something and then you excel in it.
Diani: What I saw over the last 10 years is a big revolution. The machine vision market is going up to very high speed and high resolution applications but also to the bottom where the application doesn’t need a lot of power. So I believe that there is space for everyone.
Hall: The reality is that the problems that we have today are best solved through a marriage of these different technologies. For example, if we want to take novel data from any camera from any geographic region, push it back up to the cloud, and to develop in an iterative way a more robust machine learning model which can then be redeployed to all those devices without anybody plugging a USB stick into the camera, how do we get there? How do we work with OEM’s? How do we work with system integrators? How do we work with end customers to provide solutions like this?
Myakov: The democratization of deployment is a missing piece right now. You can train a model but putting it on a camera is complex because tho thirds of the market globally is run with a very closed ecosystem approach. So essentially there are cameras, but there’s no way for anybody to deploy on them. In machine vision the situation is different, but it’s a niche market. But if you think globally, that’s what’s gonna actually impede the progress. And I totally agree that training in the cloud and deploying anywhere you want is what’s going to drive innovation. I don’t think we have uncovered all of the use cases out there just yet. So having the ability to deploy on any device would be important and instrumental in driving that innovation, but there are some very objective barriers to that.
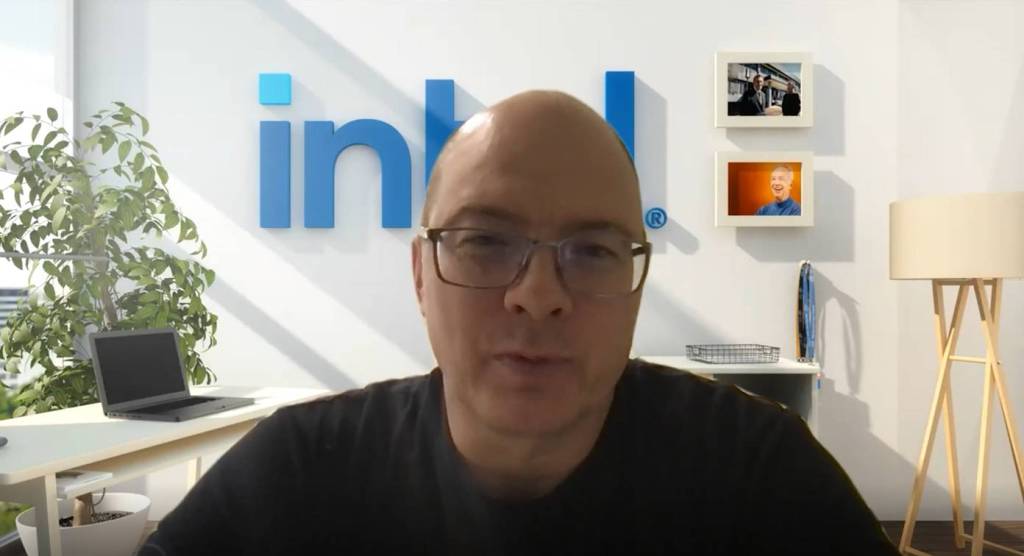
What is your best estimate as to when it will be completely the norm to store and share quality and production data via the cloud?
Metzner: This is already the norm. Therefore, if you haven’t done it yet, you need to prepare for it. There are barriers that you need to overcome. Some technical challenges, let’s say on the networking side. But it can be done. The real problem is in the mind. If you are afraid of new things, you will not change it. This is actually the barrier that we are facing most of the time.