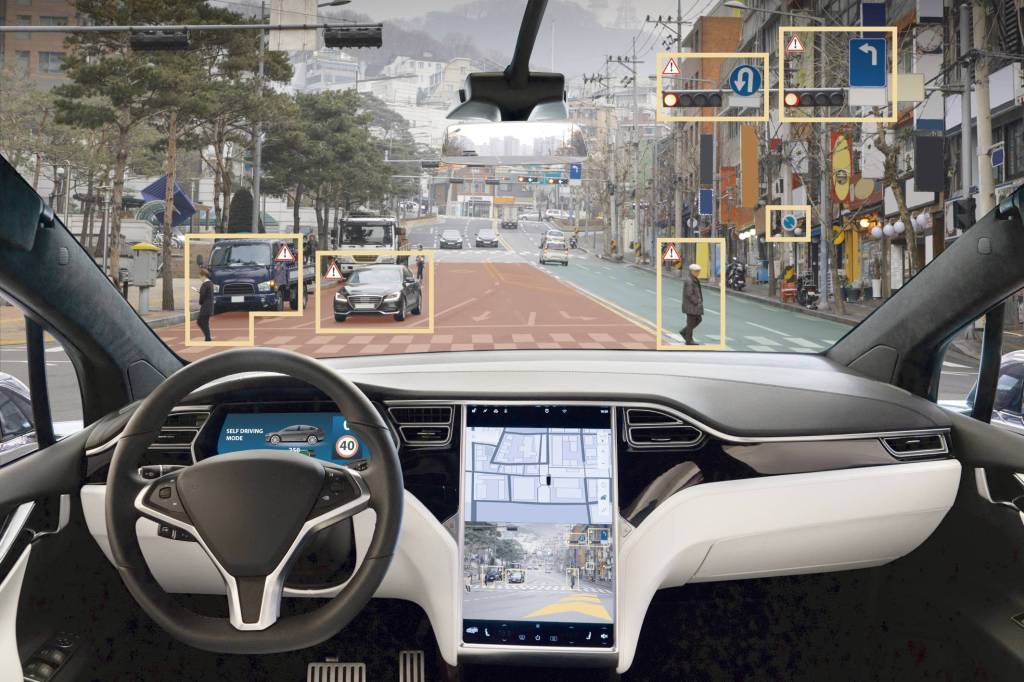
AI4IV’s intelligent vision sensors are based on two biology-inspired innovations:
- Sensing technology: a new architecture (FlyEye) optimizing the acquisition of 2D images meant to be processed using neural networks.
- Processing technology: a new approach (Tensputing) allowing the implementation in silicon´ of highly efficient neural processors with very low energy envelope/space footprint.
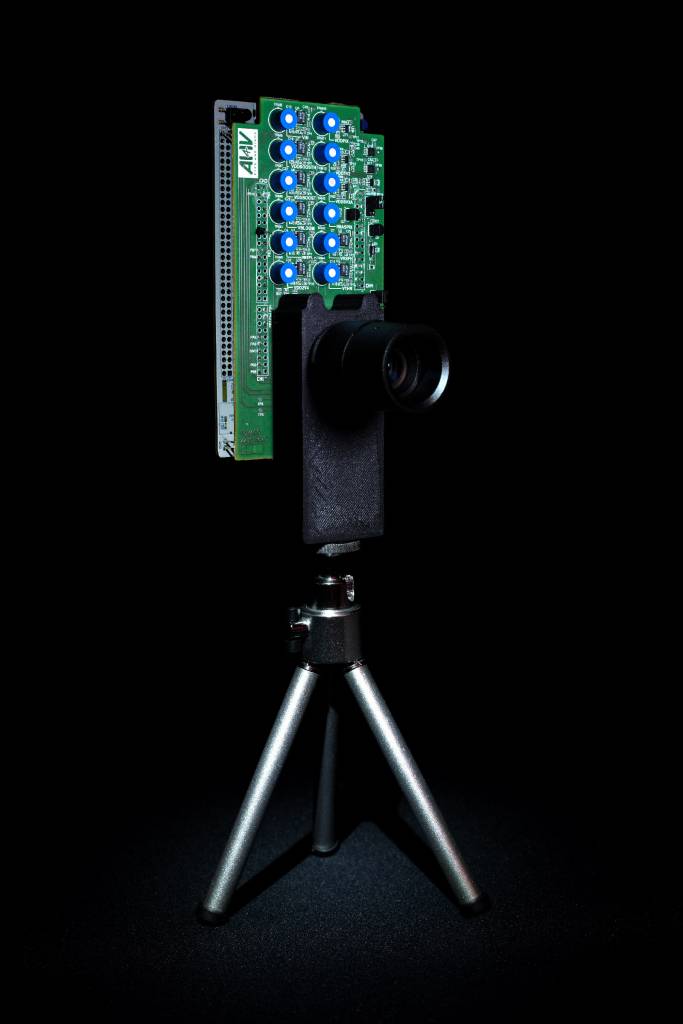
Limitations of CMOS Sensors
Current CMOS Image Sensors (CIS) cannot cope with harsh lighting variations, when a bright light source is part of a scene together with very dark areas and that can generate images with saturated regions where the information content is hidden. Post-processing techniques (like HDR) can mitigate such problem but can introduce artifacts that are invisible to humans but potentially misleading for AI systems. Hence, they can lead to misclassifications, hindering object recognition in mission-critical applications.
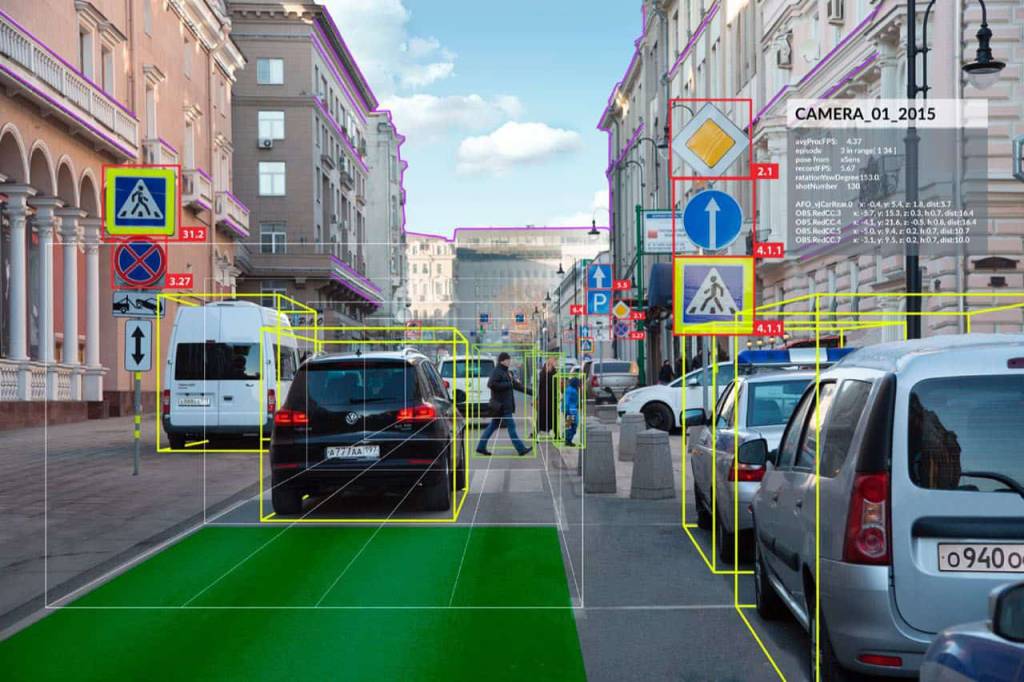
FlyEye Vision Sensor
AI4IV’s vision sensors are based on an array of photosensitive elements, each working autonomously and independently in analogy with the equivalent biological organs (eyes and brains). Each photoreceptor can optimize its response to the local light characteristics working like an independent small eye in a larger composite eye (hence the name of the architecture FlyEye). This unique approach solves the problems arising from strong light-intensity gradients in the scene without introducing artifacts because no image postprocessing is required. Images acquired by FlyEye sensors guarantee that the information content of the scene is fully preserved even in the harshest lighting conditions. Also, the architecture allows the use of different types of photoreceptors in the same sensor. For example, in an array of photoreceptors, some can be specialized in detecting moving objects so that sensors can be activated only when such objects are present in the scene. Finally, the operation of each photoreceptor can be controlled by global feedback signals generated by the neural nets integrated into the recognition stage. Such global feedback signals emulate the behavior of our brain when it sends requests to our eyes to better acquire portions of the scene or to focus our attention on specific objects.
Processing Technology
The high computational power required by Deep Neural Networks (DNNs) to classify/recognize objects and situations makes it highly challenging to integrate suitable processing devices with CMOS image sensors with a system-on-chip (SoC) or system-in-package (SoP) approach because of the power and space requirements of the processing devices. Typically, such processing units are built around the typical ´von Neumann architecture´, where the infrastructure necessary to store, read, and write the data in the memory elements requires a significant amount of silicon space. This represents a major performance bottleneck and an important source of power consumption.